- Imprimer
- Partager
- Partager sur Facebook
- Partager sur Twitter
- Partager sur LinkedIn
- Share url
Séminaire
Le 5 octobre 2023
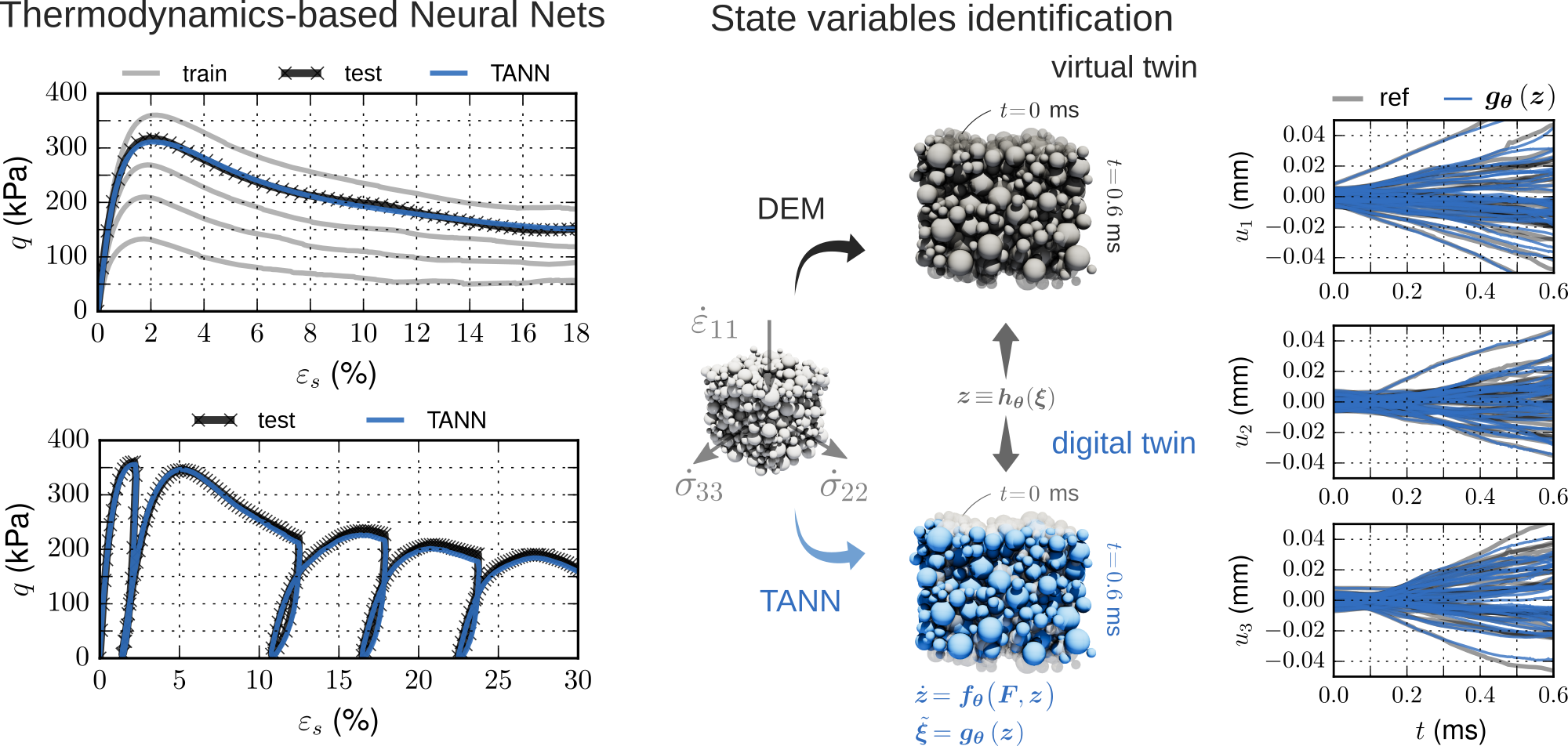
Discovery of neural constitutive equations of complex materials
Accurate models for the behaviour of materials are of fundamental importance in material science and mechanics. Traditionally, these models are derived from first principles (thermodynamics) and fine-tuned using heuristic/empirical methods to ensure calibration over experiments. However, heuristic constitutive modelling can fall short in describing the behaviour of complex materials that display path-dependent behaviours and possess multiple inherent scales, e.g. metamaterials, geomaterials, and biomaterials.
In recent years, the advent of Machine Learning, fuelled by a continuously increasing flow of data, has provided promising solutions to address the limitations of traditional constitutive modelling. Here, we present the Thermodynamics-based Artificial Neural Networks (Masi et al. 2021; Masi and Stefanou, 2022), which embed the fundamental laws of thermodynamics directly into their structure, thus ensure thermodynamically consistent predictions.
This talk mainly focuses on two major issues: (1) the non trivial identification of representative material state variables (Masi and Stefanou, 2023)—an essential ingredient in non-equilibrium thermodynamics—and (2) the shortcoming of ML in dealing with small data, i.e. limited and sparse material data sets (Masi and Einav, 2023). The capabilities of the methodology are demonstrated for the constitutive modelling of several complex, multiscale materials, displaying inelastic behaviour, path- and rate-dependency.
In recent years, the advent of Machine Learning, fuelled by a continuously increasing flow of data, has provided promising solutions to address the limitations of traditional constitutive modelling. Here, we present the Thermodynamics-based Artificial Neural Networks (Masi et al. 2021; Masi and Stefanou, 2022), which embed the fundamental laws of thermodynamics directly into their structure, thus ensure thermodynamically consistent predictions.
This talk mainly focuses on two major issues: (1) the non trivial identification of representative material state variables (Masi and Stefanou, 2023)—an essential ingredient in non-equilibrium thermodynamics—and (2) the shortcoming of ML in dealing with small data, i.e. limited and sparse material data sets (Masi and Einav, 2023). The capabilities of the methodology are demonstrated for the constitutive modelling of several complex, multiscale materials, displaying inelastic behaviour, path- and rate-dependency.
- F. Masi, I. Stefanou, P. Vannucci, V. Maffi-Berthier (2021). Thermodynamics-based Artificial Neural Networks for constitutive modeling. J Mech Phys Solids 147, 104277. doi: 10.1016/j.jmps.2020.104277.
- F. Masi, I. Stefanou (2022a). Multiscale modeling of inelastic materials with Thermodynamics-based Artificial Neural Networks (TANN), Comput Methods Appl Mech Eng 398, 115190. doi: 10.1016/j.cma.2022.115190.
- F. Masi, I. Stefanou (2023). Evolution TANN and the identification of internal variables and evolution
- equations in solid mechanics, J Mech Phys Solids 174, 105245. doi: 10.1016/j.jmps.2023.105245.
- F. Masi, I. Einav (2023). Neural differential constitutive equations for small data. Under preparation.
Date
Le 5 octobre 2023
Complément date
at 10h00
Localisation
Complément lieu
Galilée room 010
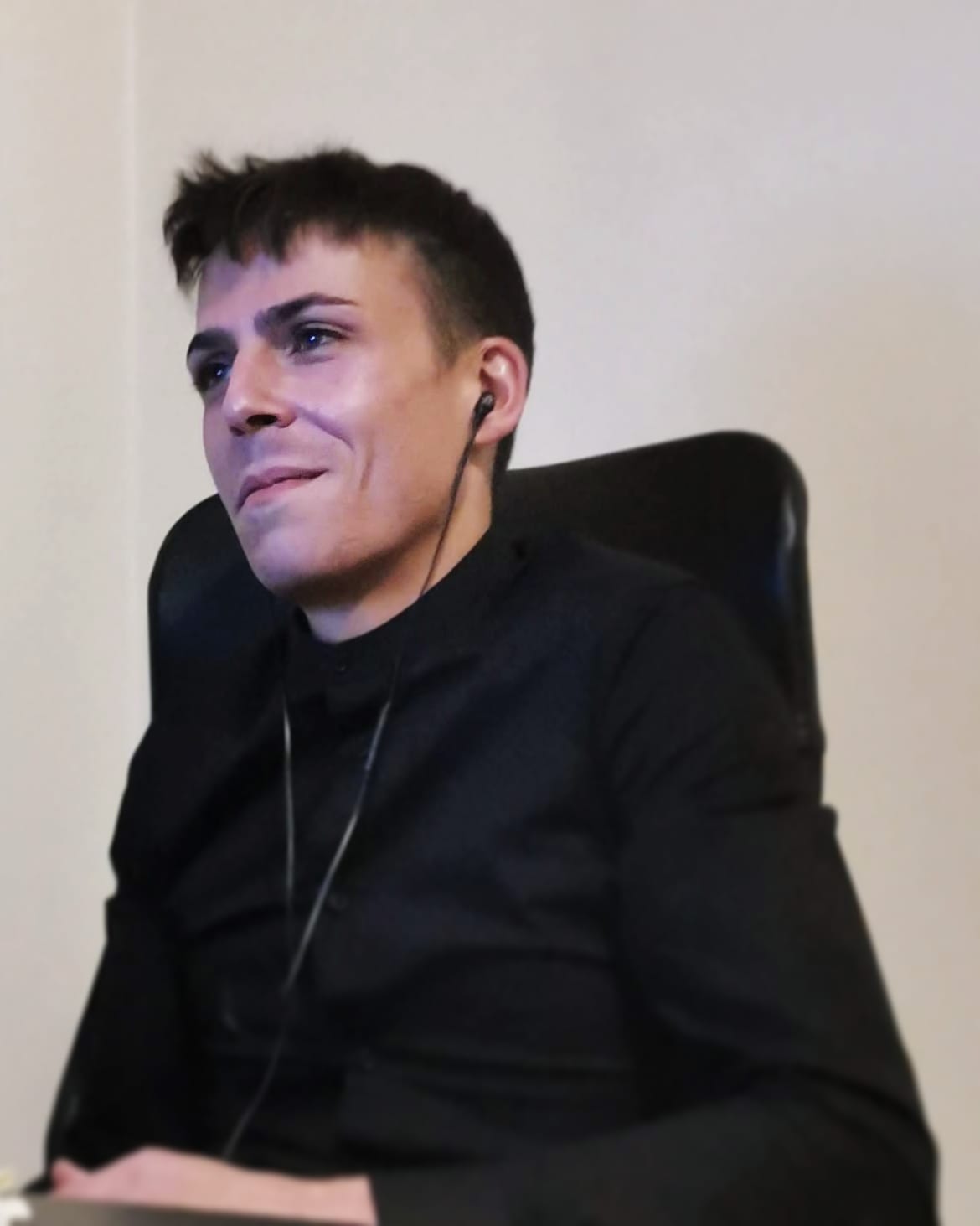
- Imprimer
- Partager
- Partager sur Facebook
- Partager sur Twitter
- Partager sur LinkedIn
- Share url