- Share
- Share on Facebook
- Share on X
- Share on LinkedIn
Séminaire
On April 29, 2021
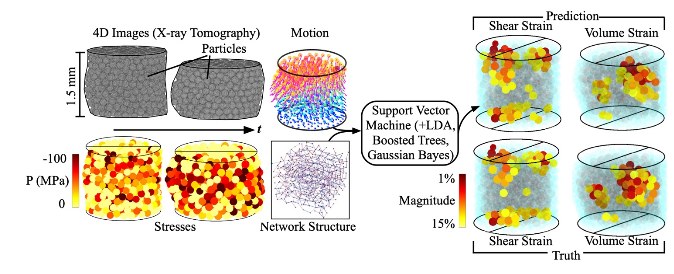
Quantifying the Causes of Local Rearrangements in 3D Granular Media Using Machine Learning
Granular materials deform macroscopically via local slip and coordinated particle rearrangement events at the microscale. Discrete and continuum numerical models have been employed in the engineering and physics communities to capture the effects of individual particle rearrangements on the macroscopic plasticity of granular and related materials. For instance, glassy rheology models and shear transformation zone theories have been used to capture the aggregated effects of local individual rearrangement events and their interactions in colloids, metallic glasses, and granular media. A major challenge remains the quantitative validation and calibration of these models using in-situ 3D experimental data.
In this talk, we discuss recent experiments combining in-situ X-ray computed tomography (XRCT) and 3D X-ray diffraction (3DXRD) to quantify local rearrangements in deforming 3D granular materials. We focus on granular materials with hundreds to thousands of nearly-spherical sapphire or quartz particles in uniaxial, hydrostatic, and triaxial loading conditions. Using microscopic structure and per-particle stress tensor measurements at small macroscopic strain increments, we examine the statistics and history-dependence of local rearrangement events, and study the features of the structure and force network that control when and where rearrangements occur via machine learning. We find that local rearrangements obey similar statistical distributions in various loading conditions and at various stress states when properly normalized, that materials retain significant memory of local rearrangements during monotonic loading, and that local structure rather than local stress plays a dominant role in predicting when and where large rearrangement events will occur. We also discuss other results from analysis of these datasets, including a hierarchical ordering of structural and mechanical length scales.
In this talk, we discuss recent experiments combining in-situ X-ray computed tomography (XRCT) and 3D X-ray diffraction (3DXRD) to quantify local rearrangements in deforming 3D granular materials. We focus on granular materials with hundreds to thousands of nearly-spherical sapphire or quartz particles in uniaxial, hydrostatic, and triaxial loading conditions. Using microscopic structure and per-particle stress tensor measurements at small macroscopic strain increments, we examine the statistics and history-dependence of local rearrangement events, and study the features of the structure and force network that control when and where rearrangements occur via machine learning. We find that local rearrangements obey similar statistical distributions in various loading conditions and at various stress states when properly normalized, that materials retain significant memory of local rearrangements during monotonic loading, and that local structure rather than local stress plays a dominant role in predicting when and where large rearrangement events will occur. We also discuss other results from analysis of these datasets, including a hierarchical ordering of structural and mechanical length scales.
Date
On April 29, 2021
Complément date
16h00
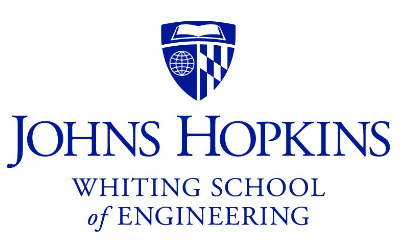
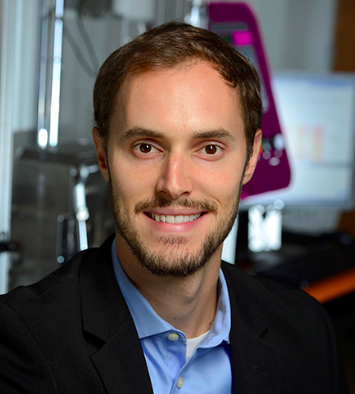
- Share
- Share on Facebook
- Share on X
- Share on LinkedIn